Mozilla, EleutherAI publish research on open datasets for LLM training
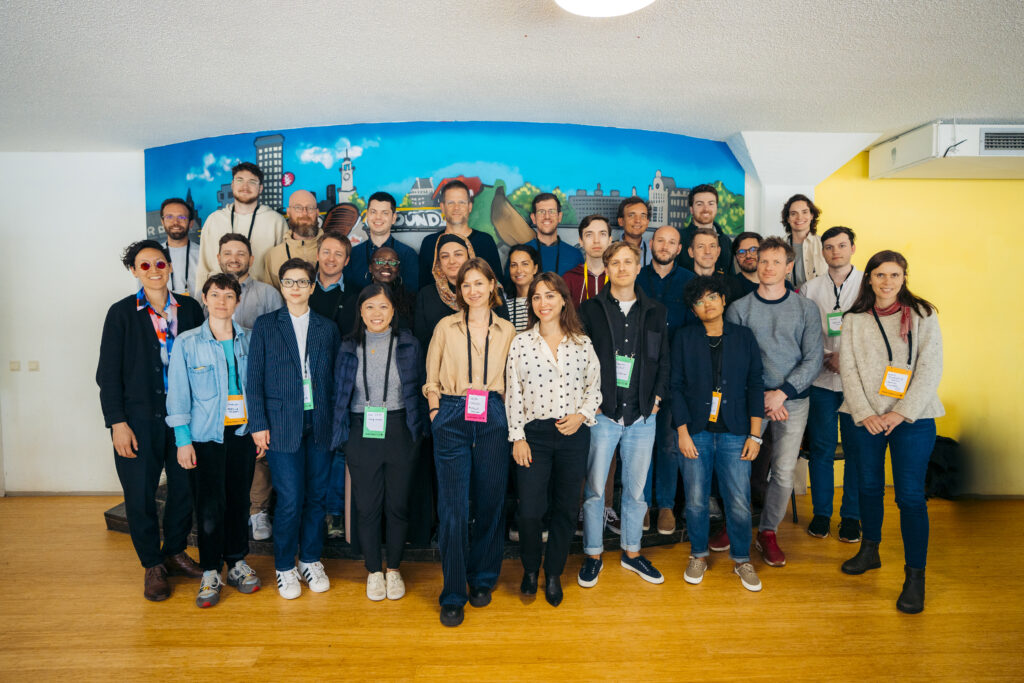
Update: Following the 2024 Mozilla AI Dataset Convening, AI builders and researchers publish best practices for creating open datasets for LLM training.
Training datasets behind large language models (LLMs) often lack transparency, a research paper published by Mozilla and EleutherAI explores how openly licensed datasets that are responsibly curated and governed can make the AI ecosystem more equitable. The study is co-authored with thirty leading scholars and practitioners from prominent open source AI startups, nonprofit AI labs, and civil society organizations who attended the Dataset Convening on open AI datasets in June 2024.
Many AI companies rely on data crawled from the web, frequently without the explicit permission of copyright holders. While some jurisdictions like the EU and Japan permit this under specific conditions, the legal landscape in the United States remains murky. This lack of clarity has led to lawsuits and a trend toward secrecy in dataset practices—stifling transparency, accountability, and limiting innovation to those who can afford it.
For AI to truly benefit society, it must be built on foundations of transparency, fairness, and accountability—starting with the most foundational building block that powers it: data.
The research, “Towards Best Practices for Open Datasets for LLM Training,” outlines possible tiers of openness, normative principles, and technical best practices for sourcing, processing, governing, and releasing open datasets for LLM training, as well as opportunities for policy and technical investments to help the emerging community overcome its challenges.
Building toward a responsible AI future requires collaboration across legal, technical, and policy domains, along with investments in metadata standards, digitization, and fostering a culture of openness.
To help advance the field, the paper compiles best practices for LLM builders, including guidance on Encoding preferences in metadata, Data sourcing, Data Processing, Data Governance/Release, and Terms of Use.
To explore the recommendations check the full paper (also available on arXiv).
We are grateful to our collaborators – 273 Ventures, Ada Lovelace Institute, Alan Turing Institute, Cohere For AI, Common Voice, Creative Commons, Data Nutrition Project, Data Provenance Initiative, First Languages AI Reality (Mila), Gretel, HuggingFace, LLM360, Library Innovation Lab (Harvard), Open Future, Pleias, Spawning, The Distributed AI Research Institute, Together AI, and Ushahidi– for their leadership in this work, as well as Computer Says Maybe for their facilitation support.
We look forward to the conversations it will spark.
Previous post published on July 2, 2024:
Mozilla and EleutherAI brought together experts to discuss a critical question: How do we create openly licensed and open-access LLM training datasets and how do we tackle the challenges faced by their builders?
On June 11, on the eve of MozFest House in Amsterdam, Mozilla and EleutherAI convened an exclusive group of 30 leading scholars and practitioners from prominent open-source AI startups, nonprofit AI labs and civil society organizations to discuss emerging practices for a new focus within the open LLM community: creating open-access and openly licensed LLM training datasets.
This work is timely. Although sharing training datasets was once common practice among many AI actors, increased competitive pressures and legal risks have made it almost unheard of nowadays for pre-training datasets to be shared or even described by their developers. However, just as open-source software has made the internet safer and more robust, we at Mozilla and EleutherAI believe open-access data is a public good that can empower developers worldwide to build upon each other’s work. It fosters competition, innovation and transparency, providing clarity around legal standing and an ability to stand up to scrutiny.
Leading AI companies want us to believe that training performant LLMs without copyrighted material is impossible. We refuse to believe this. An emerging ecosystem of open LLM developers have created LLM training datasets —such as Common Corpus, YouTube-Commons, Fine Web, Dolma, Aya, Red Pajama and many more—that could provide blueprints for more transparent and responsible AI progress. We were excited to invite many of them to join us in Amsterdam for a series of discussions about the challenges and opportunities of building an alternative to the current status quo that is open, legally compliant and just.
During the event, we drew on the learnings from assembling “Common Pile” (the soon-to-be-released dataset by EleutherAI composed only of openly licensed and public domain data) which incorporates many learnings from its hugely successful predecessor, “The Pile.” At the event, EleutherAI released a technical briefing and an invitation to public consultation on Common Pile.

Our goal with the convening was to bring in the experiences of open dataset builders to develop normative and technical recommendations and best practices around openly licensed and open-access datasets. Below are some highlights of our discussion:
- Openness alone does not guarantee legal compliance or ethical outcomes, we asked which decision points can contribute to datasets being more just and sustainable in terms of public good and data rights.
- We discussed what “good” looks like, what we want to avoid, what is realistic and what is already being implemented in the realm of sourcing, curating, governing and releasing open training datasets.
- Issues such as the cumbersome nature of sourcing public domain and openly licensed data (e.g. extracting text from PDFs), manual verification of metadata, legal status of data across jurisdictions, retractability of consent, preference signaling, reproducibility and data curation and filtering were recurring themes in almost every discussion.
- To enable more builders to develop open datasets and unblock the ecosystem, we need financial sustainability and smart infrastructural investments that can unblock the ecosystem.
- The challenges faced by open datasets today bear a resemblance to those encountered in the early days of open source software (data quality, standardization and sustainability). Back then, it was the common artifacts that united the community and provided some shared understanding and language. We saw the Dataset Convening as an opportunity to start exactly there and create shared reference points that, even if not perfect, will guide us in a common direction.
- The final insight round underscored that we have much to learn from each other: we are still in the early days of solving this immense challenge, and this nascent community needs to collaborate and think in radical and bold ways.

We are immensely grateful to the participants in the Dataset Convening (including some remote contributors):
- Stefan Baack — Researcher and Data Analyst, Insights, Mozilla
- Mitchell Baker — Chairwoman, Mozilla Foundation
- Ayah Bdeir — Senior Advisor, Mozilla
- Julie Belião — Senior Director of Product Innovation, Mozilla.ai
- Jillian Bommarito — Chief Risk Officer, 273 Ventures
- Kasia Chmielinski — Project Lead, Data Nutrition Project
- Jennifer Ding — Senior Researcher, Alan Turing Institute
- Alix Dunn — CEO, Computer Says Maybe
- Marzieh Fadaee — Senior Research Scientist, Cohere For AI
- Maximilian Gahntz — AI Policy Lead, Mozilla
- Paul Keller — Director of Policy and Co-Founder, Open Future
- Hynek Kydlíček — Machine Learning Engineer, HuggingFace
- Pierre-Carl Langlais — Co-Founder, Pleias
- Greg Leppert — Director of Product and Research, the Library Innovation Lab, Harvard
- EM Lewis-Jong — Director, Common Voice, Mozilla
- Shayne Longpre — Project Lead, Data Provenance Initiative
- Angela Lungati — Executive Director, Ushahidi
- Sebastian Majstorovic — Open Data Specialist, EleutherAI
- Cullen Miller — Vice President of Policy, Spawning
- Victor Miller — Senior Product Manager, LLM360
- Kasia Odrozek — Director, Insights, Mozilla
- Guilherme Penedo — Machine Learning Research Engineer, HuggingFace
- Neha Ravella — Research Project Manager, Insights Mozilla
- Michael Running Wolf — Co-Founder and Lead Architect, First Languages AI Reality, Mila
- Max Ryabinin — Distinguished Research Scientist, Together AI
- Kat Siminyu — Researcher, The Distributed AI Research Institute
- Aviya Skowron — Head of Policy and Ethics, EleutherAI
- Andrew Strait — Associate Director, Ada Lovelace Institute
- Mark Surman — President, Mozilla Foundation
- Anna Tumadóttir — CEO, Creative Commons
- Marteen Van Segbroeck — Head of Applied Science, Gretel
- Leandro von Werra — Chief Loss Officer, HuggingFace
- Maurice Weber — AI Researcher, Together AI
- Lee White — Senior Full Stack Developer, Ushahidi
- Thomas Wolf — Chief Science Officer and Co-Founder, HuggingFace
In the coming weeks, we will be working with the participants to develop common artifacts that will be released to the community, along with an accompanying paper. These resources will help researchers and practitioners navigate the definitional and executional complexities of advancing open-access and openly licensed datasets and strengthen the sense of community.
The event was part of the Mozilla Convening Series, where we bring together leading innovators in open source AI to tackle thorny issues and help move the community and movement forward. Our first convening was the Columbia Convening where we invited 40 leading scholars and practitioners to develop a framework for defining what openness means in AI. We are committed to continuing the efforts to support communities invested in openness around AI and look forward to helping grow and strengthen this movement.